In today's fast-paced and ever-evolving retail industry, staying ahead of the competition is crucial for success. Retailers face the challenge of understanding their customers' preferences, optimizing their operations, and driving growth in a highly dynamic marketplace. This is where the power of data analytics comes into play. By harnessing the vast amount of data generated in the retail ecosystem, businesses can unlock valuable insights and transform them into profitable opportunities. In this article, we explore the fascinating realm of retail analytics strategies that drive growth and demonstrate how data can be transformed into dollars.
Gone are the days when gut instincts and guesswork formed the basis of retail decision-making. In the digital era, data reigns supreme. Every customer interaction, purchase, and browsing session leaves a digital footprint, providing retailers with a treasure trove of information waiting to be tapped into. Retail analytics offers the tools and methodologies to collect, analyze, and interpret this wealth of data, enabling businesses to make informed decisions based on actionable insights. By understanding customer behavior, market trends, and operational inefficiencies, retailers can implement targeted strategies that drive growth and boost profitability.
The retail landscape has witnessed a seismic shift in recent years, with online channels, e-commerce platforms, and mobile applications becoming integral to the shopping experience. This transformation has resulted in an explosion of data, presenting both a challenge and an opportunity for retailers. However, collecting vast amounts of data is not enough; it is the strategic utilization of that data that holds the key to success. By employing sophisticated analytics strategies, retailers can extract meaningful patterns, identify opportunities for improvement, and deliver personalized experiences to their customers. From optimizing product assortments and pricing strategies to enhancing supply chain efficiency and customer retention, data-driven insights can propel businesses forward in the quest for growth.
In a world where customer expectations are constantly evolving, retail analytics offers a competitive edge by enabling retailers to stay one step ahead. By leveraging advanced analytics techniques such as predictive modeling, machine learning, and artificial intelligence, businesses can uncover hidden patterns and make accurate forecasts, driving better decision-making and minimizing risks. Moreover, retail analytics allows retailers to understand the impact of marketing campaigns, identify the most profitable customer segments, and tailor their offerings to meet specific demands. In essence, it empowers businesses to align their strategies with customer preferences, enhance operational efficiency, and ultimately convert data into dollars.
Importance of data-driven decisions in the retail industry

Data-driven decisions have become increasingly crucial in the retail industry due to the rapidly changing business landscape and evolving customer expectations. In an era where technology and digital advancements have disrupted traditional retail models, retailers must adapt and stay ahead of the curve to remain competitive. Data-driven decision-making provides the means to gain deep insights into customer behavior, market trends, and operational performance.
One of the key advantages of data-driven decisions is the ability to understand customers on a granular level. By analyzing customer data, retailers can uncover valuable information about purchasing patterns, preferences, and demographics. This knowledge allows retailers to personalize marketing strategies, tailor product offerings, and optimize pricing to meet the specific needs of their target audience. It enables retailers to create compelling customer experiences that build loyalty and drive repeat business.
Furthermore, data-driven decisions help retailers identify emerging trends and adapt their strategies accordingly. By analyzing market data, retailers can spot shifts in consumer preferences, anticipate demand fluctuations, and adjust their inventory and assortment planning accordingly. This agility enables retailers to stay ahead of the competition, introduce innovative products, and capture new market opportunities.
Operational efficiency is another area where data-driven decisions play a vital role. Retailers can leverage data to optimize supply chain management, streamline inventory management, and enhance operational processes. By analyzing data on sales, stock levels, and customer demand, retailers can make informed decisions about procurement, distribution, and logistics, ensuring that the right products are available at the right time and in the right locations. This leads to cost savings, improved customer satisfaction, and increased profitability.
Predictive Analytics in Retail: How to Use Data to Forecast Future Trends

Predictive analytics has become a game-changer in the retail industry, empowering businesses to forecast future trends and make informed decisions. By harnessing the power of data and leveraging advanced analytical techniques, retailers can gain a competitive edge and stay ahead of changing market dynamics. Here are key steps to effectively use data for forecasting future trends in retail through predictive analytics:
Data Collection and Preparation: Start by collecting and organizing relevant data from various sources, such as sales records, customer behavior data, market trends, and external factors. Ensure the data is accurate, complete, and properly formatted for analysis. Cleanse and preprocess the data to remove outliers, handle missing values, and create a consistent dataset.
Identify Key Variables and Patterns: Determine the variables that have the most significant impact on the trends you want to forecast. This may include factors like seasonality, promotional activities, economic indicators, or customer demographics. Analyze historical data to identify patterns, correlations, and relationships between these variables and the trends you want to predict.
Select Predictive Models: Choose appropriate predictive modeling techniques based on your data and objectives. Common methods include regression analysis, time series analysis, machine learning algorithms (such as decision trees, random forests, or neural networks), and forecasting models like ARIMA (Autoregressive Integrated Moving Average) or Prophet. Each model has its strengths and limitations, so select the one that aligns with your specific forecasting needs.
Train and Validate the Model: Split your historical data into training and validation sets. Use the training data to build and train the predictive model, adjusting parameters and refining the model as needed. Validate the model using the validation set to assess its accuracy and reliability. Fine-tune the model as necessary to improve its performance.
Apply the Model for Forecasting: Once you have a validated model, apply it to the most recent data to generate forecasts for future trends. Use the identified variables and patterns to input relevant data into the model and obtain predictions. Monitor the model's performance and update it periodically as new data becomes available.
Interpret and Act on the Results: Analyze the forecasts and interpret the insights derived from the predictive analytics. Understand the potential implications of the trends on your business, such as changes in customer demand, shifts in market preferences, or emerging opportunities. Incorporate the forecasts into your strategic planning, inventory management, marketing campaigns, and overall decision-making processes.
Monitor and Evaluate: Continuously monitor the accuracy of the forecasts and compare them with actual outcomes. Assess the performance of your predictive models and iterate as necessary to improve their accuracy over time. Regularly review the forecasts, update the models with new data, and refine your strategies based on the insights gained.
Understanding predictive analytics and its significance in retail
Predictive analytics is a powerful technique that utilizes historical data, statistical algorithms, and machine learning to make predictions and forecast future outcomes in the retail industry. It enables retailers to anticipate customer behavior, market trends, and business performance, empowering them to make informed decisions and gain a competitive edge. Here's why predictive analytics is significant in retail:
Anticipating Customer Demand: Predictive analytics helps retailers forecast customer demand by analyzing historical sales data, customer behavior patterns, and market trends. By understanding customer preferences, buying patterns, and seasonality effects, retailers can optimize inventory levels, plan product assortments, and ensure the availability of popular items. Anticipating customer demand leads to improved customer satisfaction, reduced stockouts, and increased sales.
Optimizing Pricing Strategies: Predictive analytics enables retailers to optimize pricing strategies by analyzing historical sales data, competitor prices, and market dynamics. By identifying price elasticity, demand patterns, and customer segmentation, retailers can determine the optimal price points for their products. This helps maximize revenue, increase profit margins, and maintain a competitive position in the market.
Enhancing Customer Personalization: Predictive analytics enables retailers to deliver personalized experiences by analyzing customer data and predicting individual preferences. By understanding customer behavior, purchase history, and demographic information, retailers can tailor marketing campaigns, recommend personalized product suggestions, and provide customized offers. This enhances customer engagement, builds loyalty, and improves the overall customer experience.
Improving Inventory Management: Predictive analytics helps retailers optimize inventory management by forecasting demand, identifying inventory gaps, and streamlining supply chain operations. By analyzing historical sales patterns, market trends, and external factors, retailers can make accurate predictions about future inventory needs, reduce excess inventory, and minimize stockouts. This improves operational efficiency, reduces costs, and ensures an optimal balance between supply and demand.
Mitigating Risk and Fraud: Predictive analytics can help retailers identify potential risks and detect fraudulent activities. By analyzing historical data, transaction patterns, and user behavior, retailers can detect anomalies, identify fraudulent transactions, and mitigate the risk of fraudulent activities. This protects the business from financial losses, safeguards customer data, and maintains brand reputation.
Driving Marketing Campaign Effectiveness: Predictive analytics empowers retailers to optimize marketing campaigns by identifying the most effective channels, messaging, and targeting strategies. By analyzing customer data, response rates, and campaign performance metrics, retailers can make data-driven decisions to allocate marketing resources effectively. This maximizes the return on investment (ROI) of marketing campaigns, increases customer engagement, and drives higher conversion rates.
Key predictive analytics techniques for retail
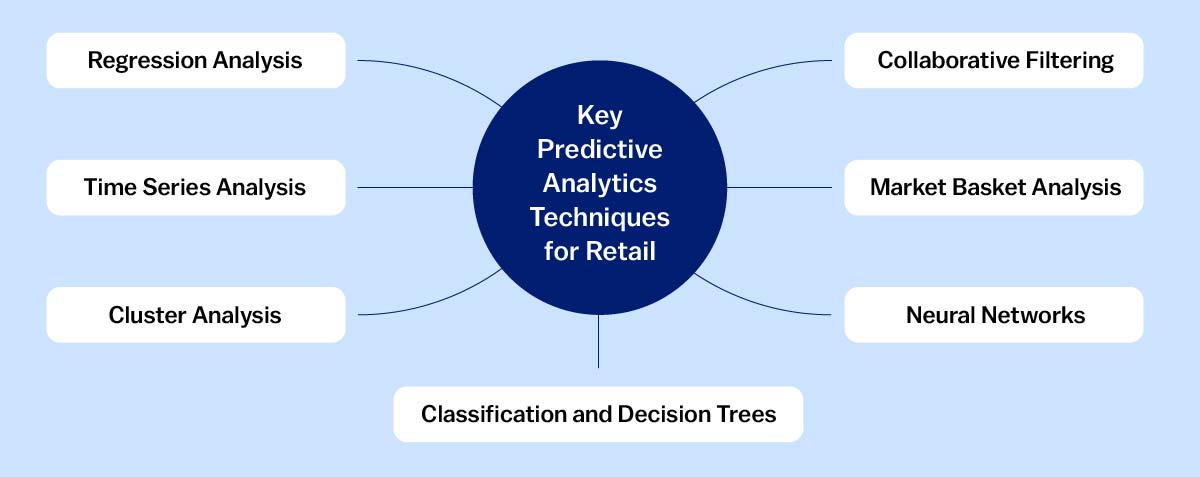
Predictive analytics techniques offer powerful tools for retailers to analyze data and make accurate predictions about future outcomes. Here are some key predictive analytics techniques commonly used in the retail industry:
Regression Analysis: Regression analysis is a statistical technique that examines the relationship between a dependent variable and one or more independent variables. In retail, regression analysis can be used to predict sales based on factors such as pricing, promotional activities, seasonality, and other relevant variables. It helps retailers understand the impact of different factors on sales and make informed decisions.
Time Series Analysis: Time series analysis involves analyzing data collected at regular time intervals to identify patterns, trends, and seasonality. Retailers often use time series analysis to forecast sales, demand, and other business metrics. Techniques like ARIMA (Autoregressive Integrated Moving Average) or exponential smoothing can be applied to time series data to make accurate predictions.
Cluster Analysis: Cluster analysis is a technique used to segment customers into distinct groups based on similarities in their behavior, preferences, or demographics. By identifying customer clusters, retailers can tailor marketing strategies, personalize product recommendations, and target specific customer segments effectively. Cluster analysis helps retailers better understand their customer base and improve customer engagement.
Classification and Decision Trees: Classification is a technique used to categorize data into predefined classes or groups based on various attributes. Decision trees, a type of classification algorithm, create a tree-like model to predict outcomes based on input variables. In retail, classification and decision trees can be used for customer segmentation, fraud detection, product categorization, and more.
Neural Networks: Neural networks are a type of machine learning technique inspired by the human brain's neural structure. They are used for pattern recognition, data classification, and prediction. In retail, neural networks can be applied to customer behavior analysis, demand forecasting, and inventory optimization. They can capture complex relationships between variables and provide accurate predictions.
Market Basket Analysis: Market basket analysis examines the relationships and associations between products purchased together by customers. It helps retailers identify product affinities and make recommendations for cross-selling and upselling opportunities. By understanding which products are commonly purchased together, retailers can optimize product placement, promotions, and inventory management.
Collaborative Filtering: Collaborative filtering is a technique used for personalized recommendations based on user behavior and preferences. By analyzing historical data, it identifies similarities between customers and recommends products or content based on what similar customers have liked or purchased. Collaborative filtering is widely used in recommendation systems for online retail platforms.
Steps to implement predictive analytics in retail for trend forecasting
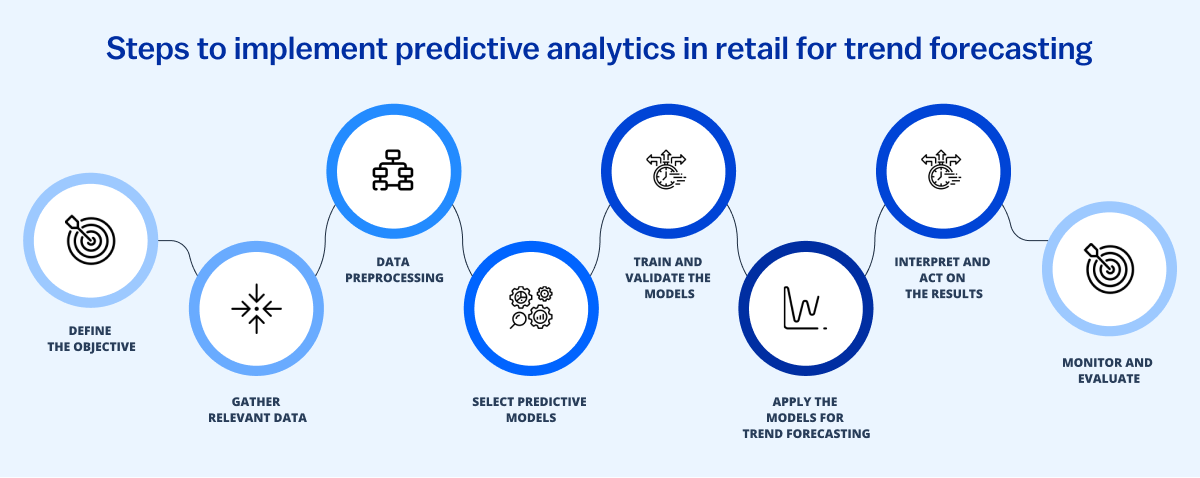
Implementing predictive analytics for trend forecasting in retail involves a systematic approach to leverage data effectively. Here are the key steps to follow:
Define the Objective: Clearly define the objective of your trend forecasting initiative. Determine the specific trends you want to predict, such as customer demand for certain products, market trends, or sales patterns. Having a well-defined objective will guide your data collection and modeling efforts.
Gather Relevant Data: Collect and compile relevant data from various sources, including historical sales data, customer behavior data, market trends, and external factors like economic indicators or weather patterns. Ensure the data is accurate, complete, and representative of the trends you want to forecast.
Data Preprocessing: Preprocess the data to ensure its quality and compatibility with the predictive modeling techniques you will employ. Cleanse the data by removing outliers, handling missing values, and normalizing variables as necessary. Prepare the data for analysis by organizing it in a structured format.
Select Predictive Models: Choose appropriate predictive modeling techniques that are suitable for trend forecasting. Common models used in retail include regression analysis, time series analysis, machine learning algorithms (such as decision trees, random forests, or neural networks), and forecasting models like ARIMA (Autoregressive Integrated Moving Average). Select the models that align with your specific objective and the nature of your data.
Train and Validate the Models: Split your historical data into training and validation sets. Use the training data to build and train the predictive models, adjusting parameters and refining the models as needed. Validate the models using the validation set to assess their accuracy and reliability. Fine-tune the models as necessary to improve their performance.
Apply the Models for Trend Forecasting: Once you have validated models, apply them to the most recent data to generate forecasts for future trends. Use the identified variables and patterns to input relevant data into the models and obtain predictions. Monitor the models' performance and update them periodically with new data.
Interpret and Act on the Results: Analyze the forecasts and interpret the insights derived from the predictive analytics. Understand the potential implications of the forecasted trends on your business and develop strategies to capitalize on opportunities or mitigate risks. Incorporate the forecasts into your strategic planning, inventory management, marketing campaigns, and overall decision-making processes.
Monitor and Evaluate: Continuously monitor the accuracy of the trend forecasts and compare them with actual outcomes. Assess the performance of your predictive models and iterate as necessary to improve their accuracy over time. Regularly review the forecasts, update the models with new data, and refine your strategies based on the insights gained.
How to Leverage Customer Data for Better Retail Analytics
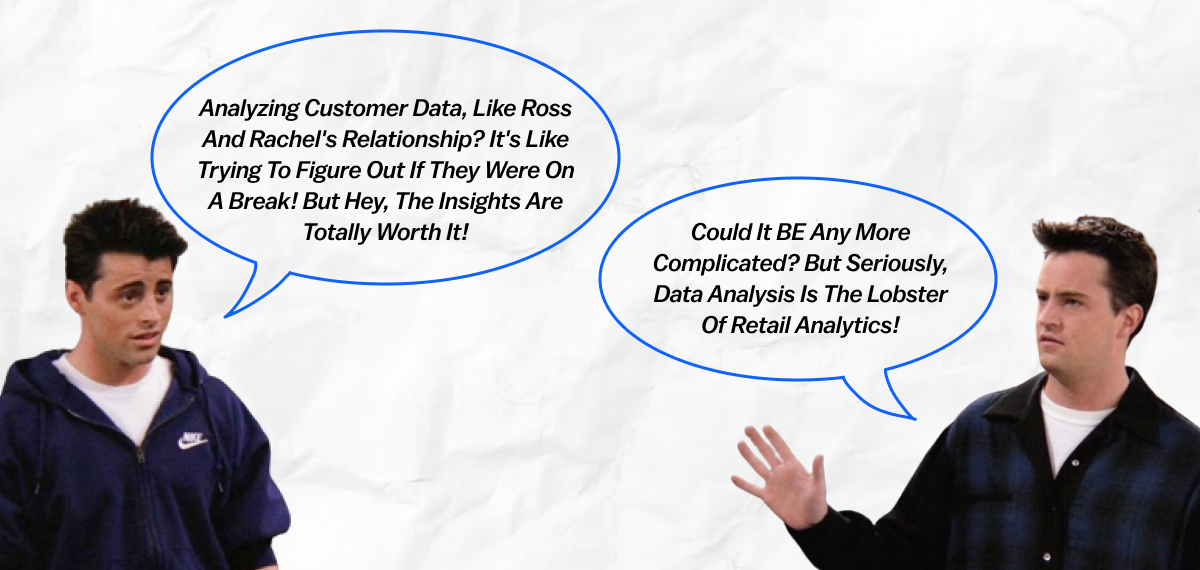
Leveraging customer data effectively is crucial for enhancing retail analytics and gaining valuable insights into customer behavior, preferences, and trends. Here are key steps to leverage customer data for better retail analytics:
Collect Comprehensive Customer Data: Gather comprehensive customer data across multiple touchpoints, such as online purchases, in-store interactions, loyalty programs, social media engagement, and customer surveys. Collect data points like demographics, purchase history, browsing behavior, product preferences, and feedback. Ensure compliance with data privacy regulations and obtain customer consent for data collection.
Integrate and Centralize Data: Integrate customer data from various sources into a centralized database or customer relationship management (CRM) system. This consolidation allows for a holistic view of customer interactions and enables analysis across different data dimensions. Cleanse and validate the data to ensure accuracy and reliability for effective analytics.
Segment Customers: Segment customers based on shared characteristics, behaviors, or preferences. Use techniques like clustering analysis or predictive modeling to identify distinct customer segments. This segmentation enables personalized targeting, tailored marketing campaigns, and specific customer-focused strategies.
Analyze Customer Behavior: Analyze customer behavior patterns to gain insights into purchasing habits, product preferences, and engagement levels. Use techniques such as RFM (Recency, Frequency, Monetary) analysis, cohort analysis, and basket analysis to understand customer value, retention, and cross-selling opportunities. Identify key trends and patterns that inform decision-making.
Personalize Customer Experiences: Leverage customer data to create personalized experiences across various touchpoints. Utilize customer insights to deliver targeted marketing messages, recommend relevant products, and personalize website or app experiences. Personalization enhances customer engagement, loyalty, and satisfaction, ultimately driving sales and customer lifetime value.
Predictive Analytics for Customer Forecasting: Apply predictive analytics techniques to forecast customer behavior and future trends. Use historical data to build predictive models that forecast customer churn, purchase intent, or lifetime value. These forecasts inform decision-making, allowing retailers to proactively address customer needs, personalize offerings, and optimize resource allocation.
Real-Time Analytics and Monitoring: Implement real-time analytics to monitor customer interactions, detect anomalies, and respond promptly to changing customer behavior. Utilize dashboards and visualizations to track key performance indicators, customer sentiment, and engagement metrics. Real-time analytics enables timely interventions and quick decision-making based on customer data insights.
Continuous Iteration and Improvement: Continuously analyze and refine customer data analytics processes. Regularly update customer profiles, evaluate the effectiveness of segmentation strategies, and fine-tune predictive models. Incorporate customer feedback and market trends into the analytics approach to ensure ongoing improvement.
Overview of customer data and its potential in retail analytics
Customer data refers to the information collected from individuals who engage with a retail business, both online and offline. It includes a wide range of data points, such as demographics, purchase history, browsing behavior, social media interactions, feedback, and more. Customer data holds immense potential in retail analytics as it provides valuable insights into customer behavior, preferences, and trends. Here's an overview of the potential of customer data in retail analytics:
Understanding Customer Behavior: Customer data allows retailers to gain a deeper understanding of how customers interact with their products, services, and channels. By analyzing data such as purchase patterns, browsing behavior, and engagement levels, retailers can identify trends, preferences, and purchase drivers. This understanding helps retailers optimize marketing strategies, improve product offerings, and enhance the overall customer experience.
Personalization and Targeted Marketing: Customer data enables retailers to deliver personalized experiences and targeted marketing campaigns. By analyzing customer demographics, purchase history, and preferences, retailers can segment their customer base and tailor marketing messages to specific customer segments. Personalization enhances customer engagement, drives higher conversion rates, and fosters long-term customer loyalty.
Customer Lifetime Value Analysis: Customer data analytics allows retailers to evaluate the lifetime value of their customers. By analyzing customer spending patterns, repeat purchase behavior, and loyalty, retailers can identify high-value customers and develop strategies to nurture and retain them. This analysis helps retailers optimize customer acquisition costs, improve customer retention, and maximize revenue from loyal customers.
Demand Forecasting and Inventory Management: Customer data is instrumental in demand forecasting and inventory management. By analyzing historical sales data, customer purchase patterns, and market trends, retailers can predict future demand and optimize inventory levels. Accurate demand forecasting ensures that retailers maintain optimal stock levels, reduce stockouts, minimize excess inventory, and improve operational efficiency.
Customer Sentiment Analysis: Customer data analytics enables retailers to gauge customer sentiment and satisfaction. By analyzing customer feedback, social media interactions, and sentiment analysis of customer reviews, retailers can gain insights into customer perceptions of products, services, or brand reputation. This analysis helps retailers identify areas for improvement, address customer concerns, and enhance overall customer satisfaction.
Cross-Selling and Upselling Opportunities: Customer data analytics identifies cross-selling and upselling opportunities by analyzing purchase history, product affinities, and customer behavior. By understanding which products are frequently purchased together or which products complement each other, retailers can tailor product recommendations and marketing strategies to increase average order value and drive additional sales.
Customer Segmentation and Personalized Experiences: Customer data allows retailers to segment their customer base into distinct groups based on shared characteristics, behaviors, or preferences. By segmenting customers, retailers can create personalized experiences, targeted offers, and customized marketing campaigns for specific customer segments. This level of personalization enhances customer engagement, satisfaction, and loyalty.
How to Identify and Address Retail Performance Issues with Analytics
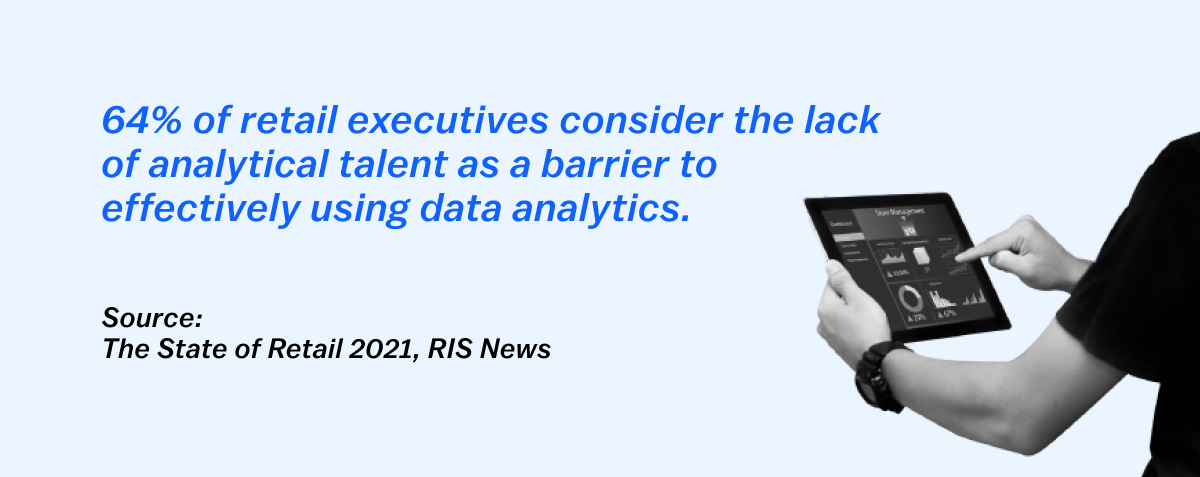
Identifying and addressing retail performance issues using analytics involves a systematic approach to analyzing data and uncovering insights. Here are key steps to effectively utilize analytics in identifying and addressing performance issues in the retail industry:
Define Key Performance Indicators (KPIs): Start by defining the KPIs that are critical to measuring retail performance. These may include metrics such as sales revenue, conversion rate, average transaction value, customer retention rate, inventory turnover, or customer satisfaction scores. Clearly define the specific KPIs that align with your business goals and objectives.
Gather Relevant Data: Collect and consolidate relevant data from various sources, such as point-of-sale systems, customer databases, website analytics, and inventory management systems. Include both internal and external data, such as sales data, customer feedback, market trends, and competitor data. Ensure data quality and accuracy to generate meaningful insights.
Analyze Data for Performance Trends: Analyze the collected data to identify performance trends and patterns. Use analytics techniques like data visualization, trend analysis, and comparative analysis to uncover insights about your retail operations. Look for anomalies, variations, or underperforming areas that may indicate performance issues.
Conduct Root Cause Analysis: Dig deeper into the data to understand the root causes of the identified performance issues. Explore factors that may contribute to the problems, such as pricing strategies, inventory management, marketing campaigns, or customer experience. Utilize statistical analysis, correlation analysis, and segmentation analysis to identify potential causes.
Utilize Predictive Analytics: Apply predictive analytics techniques to forecast future performance and identify potential issues before they occur. Use historical data and predictive modeling to anticipate trends, predict demand fluctuations, or forecast sales performance. This enables proactive measures to address performance issues before they impact the business.
Develop Actionable Insights: Based on the analysis and insights gained from the data, develop actionable insights and recommendations to address the identified performance issues. Prioritize the insights based on their potential impact on business outcomes and feasibility of implementation. Ensure the recommendations are specific, measurable, achievable, relevant, and time-bound (SMART).
Implement Performance Improvement Strategies: Execute the recommended actions to address the performance issues. This may involve adjusting pricing strategies, optimizing inventory levels, refining marketing campaigns, improving operational processes, or enhancing the customer experience. Monitor the impact of the implemented strategies and track the relevant KPIs to evaluate their effectiveness.
Continuously Monitor and Refine: Continuously monitor performance metrics and regularly analyze data to track the progress of the implemented strategies. Identify areas that require further improvement and refine the strategies as needed. Embrace a culture of continuous improvement and utilize analytics as an ongoing tool for monitoring and optimizing retail performance.
The role of analytics in identifying performance issues in retail
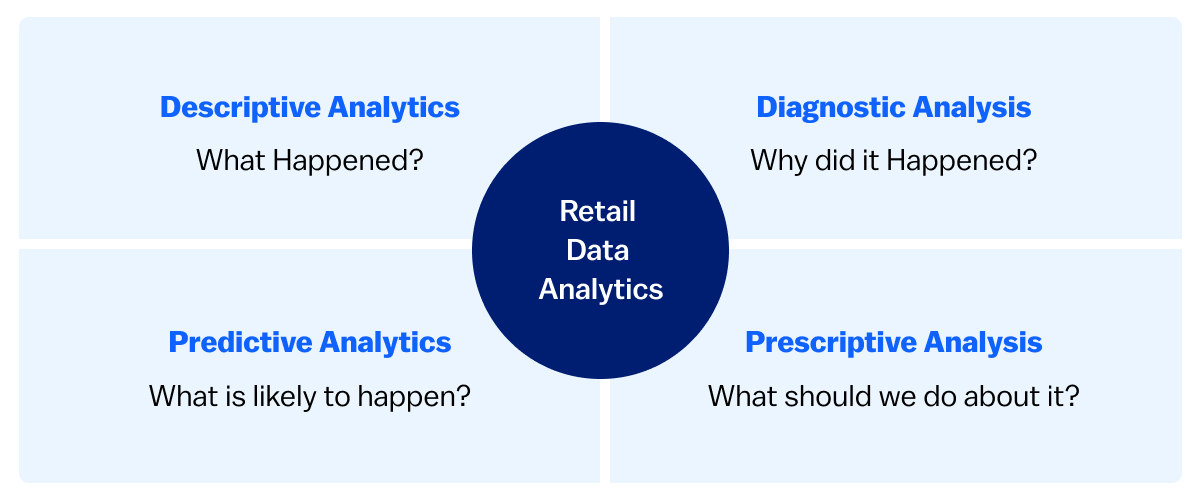
Analytics plays a critical role in identifying performance issues in the retail industry by providing insights into various aspects of business operations. Here are the key roles of analytics in identifying performance issues in retail:
Data Analysis and Visualization: Analytics enables retailers to analyze large volumes of data from multiple sources, such as sales records, customer behavior data, inventory levels, and operational metrics. By leveraging data visualization techniques, retailers can identify patterns, trends, and anomalies that may indicate performance issues. Visual representations of data make it easier to spot areas of concern and understand the overall performance of the business.
Key Performance Indicator (KPI) Tracking: Analytics allows retailers to track and monitor key performance indicators that reflect the health of their business. By setting up dashboards and reports, retailers can regularly review metrics such as sales revenue, profit margin, customer acquisition costs, inventory turnover, and customer satisfaction scores. Tracking KPIs helps identify deviations from targets and pinpoint areas that require improvement.
Comparative Analysis: Analytics enables retailers to perform comparative analysis across different time periods, store locations, product categories, or customer segments. By comparing performance metrics, retailers can identify underperforming areas or segments that need attention. Comparative analysis helps identify trends, outliers, and benchmark performance against competitors or industry standards.
Root Cause Analysis: Analytics facilitates root cause analysis by examining relationships and correlations between various factors influencing performance. By analyzing data, retailers can determine the underlying causes of performance issues, such as pricing strategies, marketing campaigns, inventory management, or customer experience. Identifying the root causes helps retailers address the core issues to improve overall performance.
Predictive Analytics: Analytics allows retailers to leverage predictive modeling techniques to forecast future performance and anticipate potential issues. By analyzing historical data and applying predictive algorithms, retailers can predict sales trends, demand fluctuations, or operational bottlenecks. Predictive analytics helps retailers take proactive measures to address performance issues before they negatively impact the business.
Customer Analytics: Analytics helps retailers analyze customer data to understand customer behavior, preferences, and satisfaction levels. By analyzing customer purchase patterns, feedback, and engagement metrics, retailers can identify areas where customer experience or service may be lacking. Customer analytics helps identify performance issues related to customer acquisition, retention, or loyalty.
Operational Efficiency Analysis: Analytics enables retailers to analyze operational data and identify inefficiencies or bottlenecks in processes. By examining data related to inventory management, supply chain operations, or workforce productivity, retailers can uncover performance issues affecting operational efficiency. This analysis helps streamline operations, reduce costs, and improve overall performance.
A/B Testing and Experimentation: Analytics supports A/B testing and experimentation, allowing retailers to test different strategies, campaigns, or operational changes. By measuring the impact of these experiments and analyzing the results, retailers can identify performance issues and make data-driven decisions to optimize their approaches.
Key performance metrics to monitor in retail analytics

In retail analytics, monitoring key performance metrics is crucial to assess the health and success of a retail business. The specific metrics that retailers should monitor may vary depending on their industry, business model, and goals. However, here are some common key performance metrics that retailers typically monitor in retail analytics:
Sales Revenue: Tracking overall sales revenue provides a clear indication of the financial performance of the business. It helps measure the effectiveness of marketing efforts, product offerings, and sales strategies.
Gross Margin: Gross margin calculates the difference between sales revenue and the cost of goods sold (COGS), expressed as a percentage. Monitoring gross margin helps retailers assess the profitability of their products and pricing strategies.
Average Transaction Value (ATV): ATV measures the average value of each customer transaction. Monitoring ATV helps retailers understand customer spending patterns and identify opportunities for upselling or cross-selling.
Conversion Rate: Conversion rate measures the percentage of visitors who make a purchase. Monitoring conversion rate helps assess the effectiveness of marketing campaigns, website design, and customer experience.
Customer Acquisition Cost (CAC): CAC measures the cost associated with acquiring each new customer. Tracking CAC helps retailers evaluate the efficiency of their marketing and sales efforts.
Customer Lifetime Value (CLV): CLV estimates the total value a customer brings to the business over their lifetime. Monitoring CLV helps retailers understand the long-term profitability of their customer base and allocate resources accordingly.
Return on Investment (ROI): ROI calculates the return on investment generated from specific marketing campaigns, initiatives, or investments. Monitoring ROI helps retailers evaluate the effectiveness and profitability of their marketing activities.
Inventory Turnover: Inventory turnover measures the rate at which inventory is sold and replenished. Monitoring inventory turnover helps retailers manage inventory levels efficiently, reduce carrying costs, and avoid stockouts or excess stock.
Customer Satisfaction Score (CSAT): CSAT measures the level of customer satisfaction with the overall experience. Monitoring CSAT helps retailers assess customer sentiment, identify areas for improvement, and track the success of customer-centric initiatives.
Churn Rate: Churn rate measures the percentage of customers who stop doing business with the company within a specific period. Monitoring churn rate helps retailers identify customer retention issues and develop strategies to improve customer loyalty.
Store Traffic and Conversion: Monitoring store footfall or website traffic provides insights into customer interest and behavior. Combining traffic data with conversion rate helps retailers optimize store layouts, staffing, and marketing strategies.
Online Engagement Metrics: Monitoring metrics such as website bounce rate, time on site, click-through rates, and social media engagement provides insights into the effectiveness of digital marketing efforts and user experience.
Strategies for addressing and improving performance issues using data
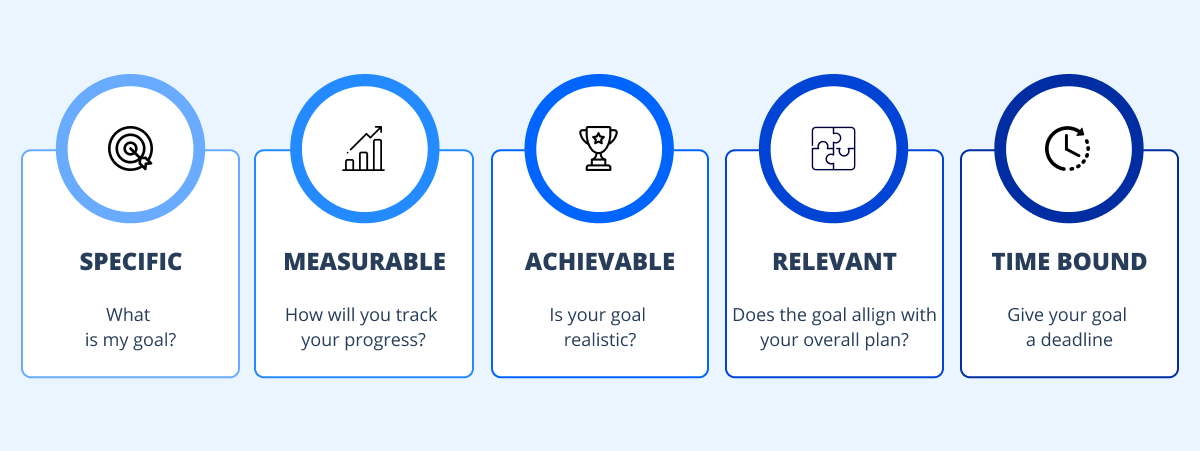
Addressing and improving performance issues using data requires strategic approaches to leverage insights and drive actionable changes. Here are some strategies for effectively utilizing data to address and improve performance issues:
Identify Performance Gaps: Analyze data to identify areas of underperformance or inefficiency within your business. This could involve examining metrics, trends, and customer feedback to pinpoint specific pain points or bottlenecks. Understand the root causes of these issues by diving deeper into the data to identify patterns, correlations, or anomalies.
Set Clear Objectives and Goals: Define specific objectives and goals to address the identified performance issues. Align these goals with the overall business strategy and ensure they are Specific, measurable, attainable, relevant, and time-bound (SMART). Clearly defined objectives provide a focus for data analysis and help track progress towards desired outcomes.
Data-Driven Decision-Making: Base decisions on data-driven insights rather than assumptions or intuition. Use data to inform strategic decisions related to pricing, inventory management, marketing campaigns, operational processes, and customer experience. Combine quantitative analysis with qualitative insights to gain a comprehensive understanding of the performance issues and potential solutions.
Establish Key Performance Indicators (KPIs): Define relevant KPIs that directly relate to the identified performance issues. KPIs provide measurable targets to track progress and evaluate the effectiveness of improvement efforts. Ensure that KPIs are aligned with the overall objectives and are specific, measurable, achievable, relevant, and time-bound (SMART).
Continuous Monitoring and Analysis: Implement systems to continuously monitor and analyze performance metrics. Regularly review and analyze the data to identify trends, changes, or anomalies that may impact performance. Implement real-time reporting and dashboards to have a pulse on the performance and address any emerging issues promptly.
Experiment and Test Solutions: Use data to drive experimentation and testing of potential solutions. Apply A/B testing or controlled experiments to evaluate different strategies or interventions. Analyze the data from these experiments to identify the most effective approaches to address the performance issues.
Collaborate Across Departments: Foster cross-functional collaboration to address performance issues holistically. Involve teams from various departments, such as marketing, operations, finance, and customer service, to collectively analyze and address the issues. Sharing insights and perspectives can lead to comprehensive solutions that consider multiple aspects of the business.
Continuous Improvement and Iteration: Embrace a culture of continuous improvement and iteration based on data insights. Regularly evaluate the effectiveness of implemented solutions and make iterative adjustments as needed. Monitor the impact of changes on performance metrics and KPIs, and refine strategies accordingly.
Employee Training and Development: Ensure that employees have the necessary skills and knowledge to leverage data effectively. Provide training and development opportunities to enhance data literacy and analytical capabilities across the organization. Empower employees to use data to make informed decisions and contribute to performance improvement efforts.
Data Governance and Data Quality: Establish robust data governance practices to ensure data accuracy, integrity, and security. Implement data quality checks, data validation processes, and data cleansing procedures to maintain reliable and trustworthy data for analysis. Data governance practices are crucial for making sound decisions and driving performance improvements.
Conclusion
In this article, we have explored the transformative potential of retail analytics strategies in driving growth and converting data into dollars. The retail industry has witnessed a paradigm shift, with data becoming the lifeblood of successful decision-making. By harnessing the power of advanced analytics techniques and leveraging the wealth of customer data available, retailers can gain a competitive edge and unlock opportunities for growth.
Retail analytics strategies offer retailers a myriad of benefits, ranging from improved customer targeting and personalized experiences to optimized inventory management and operational efficiency. By understanding customer behavior patterns, preferences, and purchasing habits, retailers can tailor their offerings, marketing campaigns, and pricing strategies to meet specific demands and maximize revenue potential.
Moreover, retail analytics empowers retailers to stay agile in a rapidly changing marketplace. By analyzing market trends, predicting future demand, and optimizing supply chain operations, businesses can mitigate risks, minimize costs, and maximize profitability. Data-driven decision-making ensures that retailers stay ahead of the competition and make strategic moves that resonate with their target audience.
As the retail industry continues to evolve, embracing retail analytics strategies becomes increasingly vital. By investing in the right tools, technologies, and talent to analyze and interpret data effectively, retailers can uncover actionable insights, make informed decisions, and drive sustainable growth.
In conclusion, the journey from data to dollars in the retail industry is fueled by the strategic implementation of retail analytics strategies. By leveraging the power of data-driven decision-making, retailers can not only survive but thrive in a competitive marketplace. The transformational potential of retail analytics is vast, offering retailers the opportunity to enhance customer experiences, optimize operations, and ultimately drive growth and profitability. It's time for retailers to embrace the data-driven revolution and unleash the true potential of their businesses.